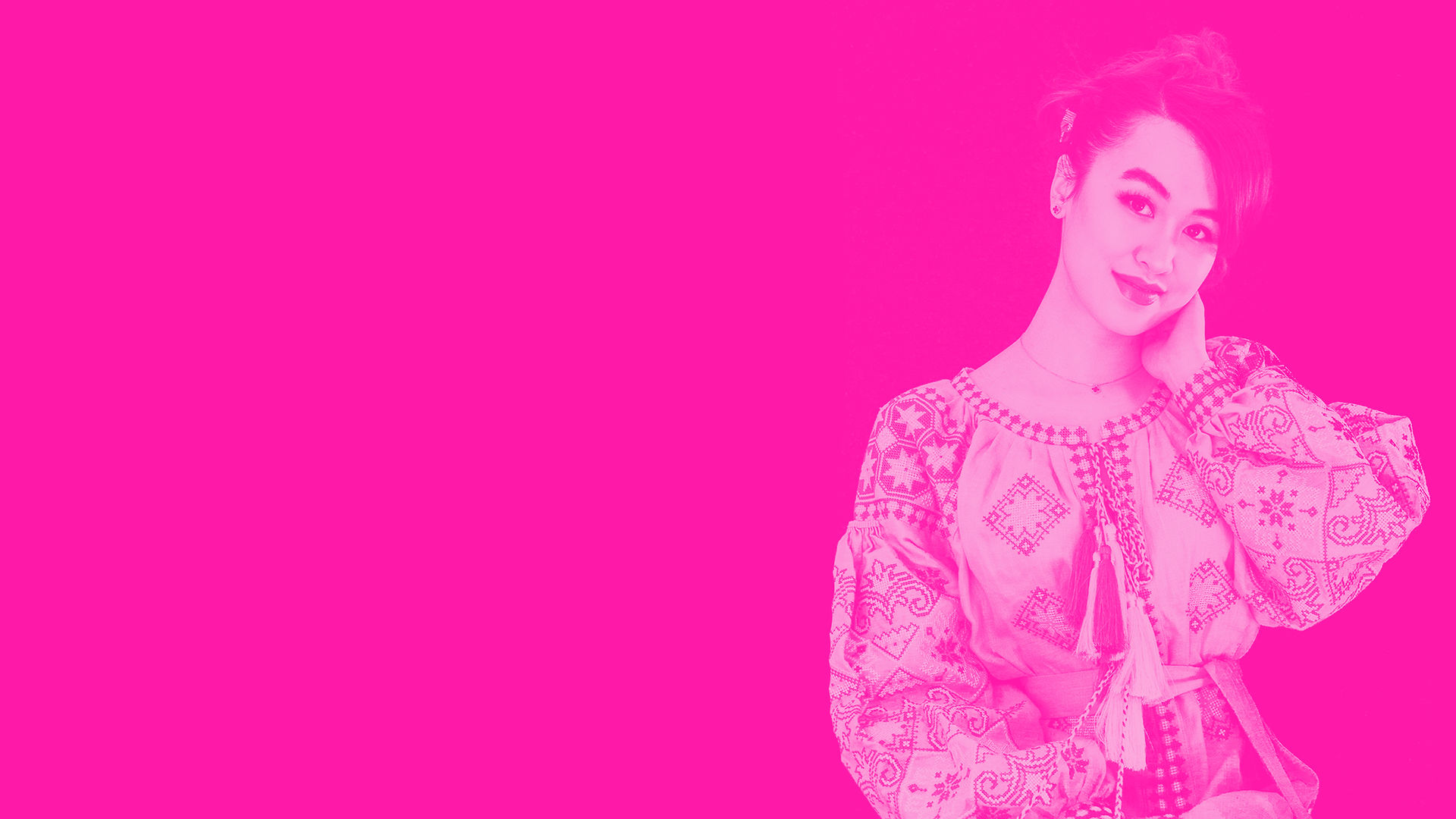

Creating Better Whole Slide Image Datasets:
Quality Control Detection of Out-Of-Focus Patches in Digital Pathology
Phoenix Wilkie [1,2]; Lukasz Itert [2]; Dina Bassiouny Abousheishaa [2]; Anne Martel [1,2]
1. Department of Medical Biophysics, University of Toronto;
2. Sunnybrook Research Institute
3 minute audio summary
Recorded T-CAIREM talk on YouTube
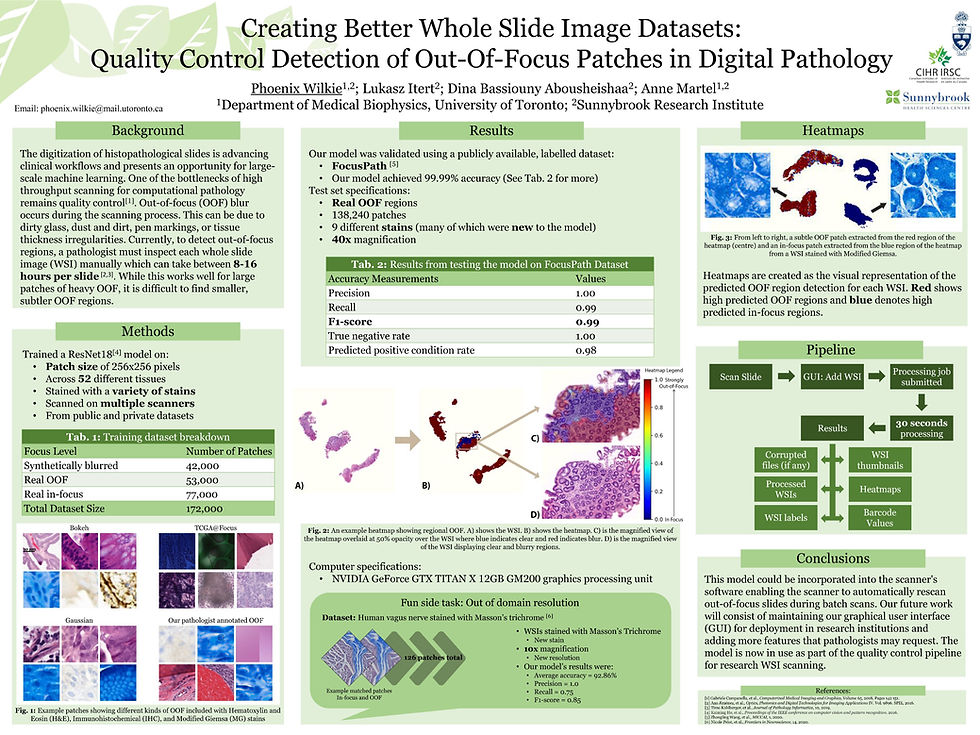
Abstract
Introduction:
The digitization of histopathological slides is advancing clinical workflows and presents an opportunity for large-scale machine learning. One of the bottlenecks of high-throughput scanning for computational pathology remains quality control. Out-of-focus (OOF) blur occurs during the scanning process. This can be due to dirty glass, dust and dirt, pen markings, or tissue thickness irregularities. Currently, to detect OOF regions, a pathologist must inspect each whole slide image (WSI) manually. This process can take 8–16 hours per slide [1]. Many pathologists also lack the coding skills to run existing, machine learning, OOF detection models without an easy-to-use graphical user interface (GUI). Therefore, we created a model with a user-friendly GUI that achieves high accuracy in finding OOF regions on commercially available computer hardware in a short amount of time.
Methods:
We have trained an accurate OOF model using WSI patches derived from 52 different tissue areas. The training dataset contains 171,436 patches with a breakdown of 41,851 synthetically blurred patches, 52,800 real OOF patches, and 76,785 clear patches. The patches were stained with different stains and taken with a variety of scanners at different resolutions. A GUI was created in FLASK for the pathologists to easily interact and employ the model.
Results:
Our model was verified using a publicly available, human-expert-labelled, dataset called FocusPath [2]. Out of 138,240 patches of 256x256 pixels, our model achieved an F-score of 0.99. A WSI can be evaluated for OOF regions in under one minute on a seven-year-old NVIDIA GeForce GTX TITAN X 12GB GM200 GPU. Model output is available as a CSV file with prediction values as well as heatmaps that can be overlaid on the original WSIs. All of these are accessible from the GUI.
Conclusion:
We propose a supervised OOF detection model packaged in a user-friendly format to improve the focus quality control pipeline for pathologists. The OOF detection model achieves high accuracy and generalizes well. The GUI is currently being used by a pathology research fellow, who reports that it greatly reduces the time they spend checking slides. This project has the potential to be integrated into built-in scanner software. This package is currently being readied for its deployment online – available for free for researchers.
References: [1] Timo Kohlberger, et al. Whole-slide image focus quality: Automatic assessment and impact on AI cancer detection. Journal of Pathology Informatics, 10, 2019. [2] Zhongling Wang, et al. FocusLiteNN: High efficiency focus quality assessment for digital pathology. 1, 2020.